To state the obvious for a moment, the potential for artificial intelligence (AI) to deliver transformational change across industries is immense. Recognizing this, forward-thinking companies are strategically planning how to best use AI to drive innovation and business impact.
But, before implementing any AI strategies, it’s important for companies to carefully consider their goals and prioritize the use cases that make the most sense. While it may be tempting to kickstart numerous small experiments to explore what works, this scattershot approach will prove to be ineffective for realizing AI’s full potential. Emerging technologies like AI evolve rapidly, and their full capabilities are still being understood. To maximize impact, companies should go deep with a focused, high-value use case before expanding broadly.
Why? Adopting disruptive, cutting-edge technology like generative AI inherently carries risks. This isn’t like making a handful of bets in a portfolio to see which one wins. AI’s novelty is its greatest strength, and its greatest weakness. Teams don’t yet know how to best use AI, so when the technology is new, it may prove best to think differently. Organizations that prematurely distribute resources across too many experimental AI projects risk being left with a graveyard of stalled initiatives. The wiser approach is to identify and concentrate efforts on mastering a single, high-impact AI use case first. Once that flagship deployment is successfully implemented and optimized, insights will quickly emerge to inform expansions into other areas.
But which use case should companies pursue first? While this decision depends on the specific business, many organizations early in their AI journey can benefit from independent AI use case research, such as Salesforce’s own publication, or McKinsey’s industry study highlighting top opportunities.
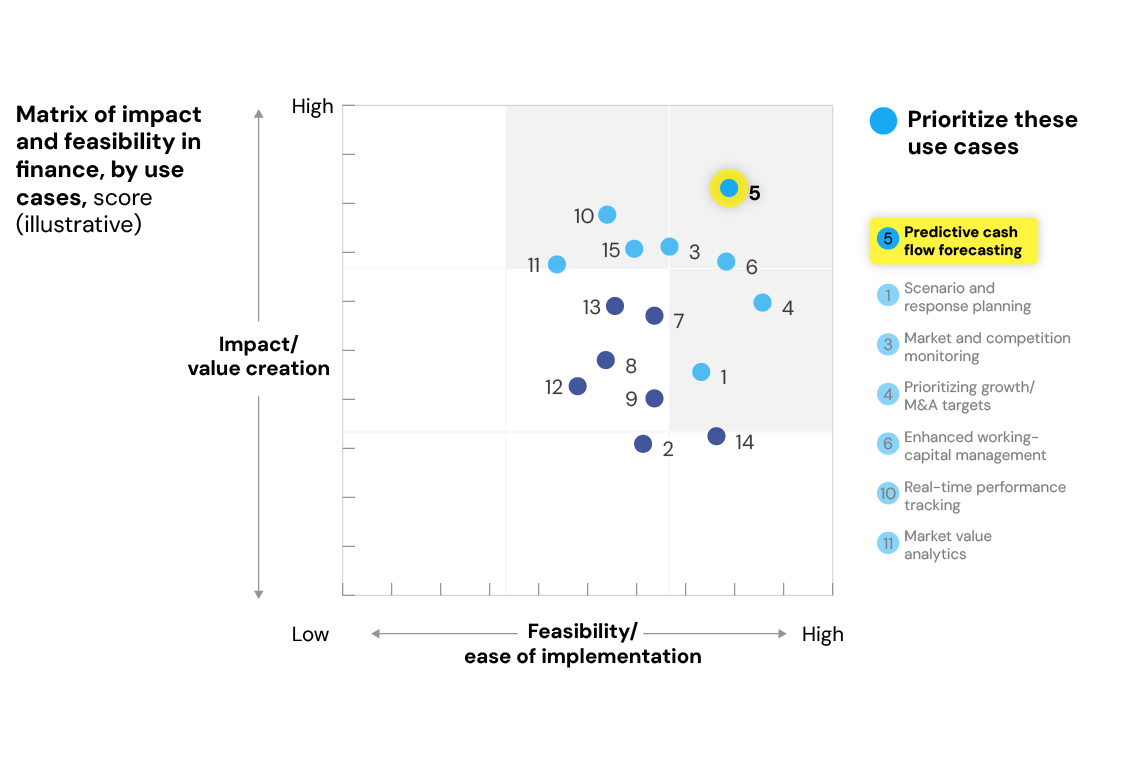
For example, McKinsey highlights finance as a prime area ripe for AI-driven transformation. This makes sense, as businesses fundamentally run on financial data like cash flow, revenue, costs, and compliance metrics. Using AI capabilities like predictive forecasting to enhance financial visibility can deliver immense value. After all, what company wouldn’t want improved ability to accurately forecast future cash positions?
Once a good initial use case is selected, the next step is ensuring the right data foundations are in place. It’s hard to overstate this. AI is data-hungry, so companies need to assess what relevant data already exists within systems like Salesforce, and identify gaps needing additional data sources.
For instance, if the chosen use case involves using AI to contextualize emails for more effective cold outreach, the company may lack sufficient data about prospect companies outside its current engagements. Having a data integration strategy to fill those contextual blind spots is critical before deploying AI in that context.
Speaking of context, the “Context Is King” adage rings true across AI use cases – from simple conversational assistants to complex enterprise deployments interacting with large language models via APIs. However, contextual data rarely materializes automatically (or economically) in real-time. While tools like ChatGPT can browse the internet ad hoc, that level of data gathering isn’t robust enough for powering scalable, commercial-grade AI applications.
We’ll explore this more in our next entry, but consider what goals you think AI could help you achieve in the near term – do they sound similar to the ones outlined above? We’d love to hear from you, so don’t hesitate to reach out to us at any time.
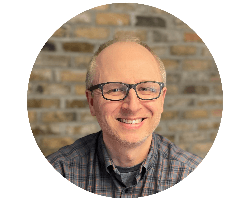
About the Author
Curt Hopmann | Founder, FreshFocus | Advisor, Breadwinner
FreshFocus is a go-to-market advisory firm for technology companies, specialized in top of funnel core message development and bottom of funnel sales execution.